NAACL
#NeuralNetwork#NLP#Chain-of-Thought#Prompting#AutomaticPromptEngineering
Issue Date: 2023-04-25 Enhancing LLM Chain-of-Thought with Iterative Bootstrapping, Sun+, Xiamen University (w_ MSRA et al.), NAACL24 CommentZero shot CoTからスタートし、正しく問題に回答できるようにreasoningを改善するようにpromptをreviseし続けるループを回す。最終的にループした結果を要約し、それらをプールする。テストセットに対しては、プールの中からNshotをサンプルしinferenceを行う。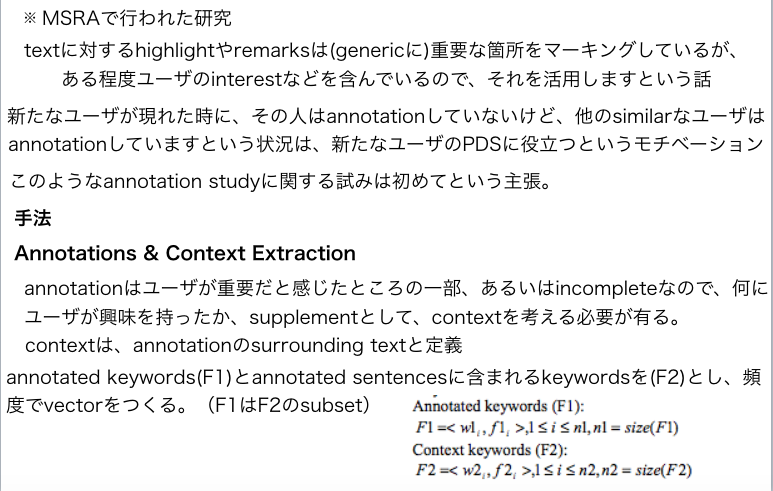 とランキング(pairwise learning-to-rank)という異なる操作が必要なタスクを、multi task learningの枠組みで ... #NaturalLanguageGeneration#SingleFramework#NLP#ConceptToTextGeneration
Issue Date: 2017-12-31 Unsupervised concept-to-text generation with hypergraphs, Konstas+, NAACL-HLT12 #NaturalLanguageGeneration#DataDriven#NLP#ConceptToTextGeneration
Issue Date: 2017-12-31 Aggregation via set partitioning for natural language generation, Barzilay+, HLT-NAACL06 #DocumentSummarization#Document#NLP
Issue Date: 2018-01-21 Cut and paste based text summarization, Jing+, NAACL00 CommentAbstractiveなSummarizationの先駆け的研究。 AbstractiveなSummarizationを研究するなら、押さえておいたほうが良い。 ... #Article#NeuralNetwork#NaturalLanguageGeneration#NLP#DataToTextGeneration
Issue Date: 2017-12-31 What to talk about and how? Selective Generation using LSTMs with Coarse-to-Fine Alignment, Mei+, NAACL-HLT’16 Commentcontent-selectionとsurface realizationをencoder-decoder alignerを用いて同時に解いたという話。 普通のAttention basedなモデルにRefinerとPre-Selectorと呼ばれる機構を追加。通常のattentionにはatte ... #Article#DocumentSummarization#NLP#IntegerLinearProgramming (ILP)#Update
Issue Date: 2017-12-28 Improving Update Summarization via Supervised ILP and Sentence Reranking, Li et al. NAACL’15, 2015.05 Comment・update summarizationをILPで定式化.基本的なMDSのILPのterm weightingにsalienceの要素に加えてnoveltyの要素を加える.term weightingにはbigramを用いる.bigram使うとよくなることがupdate summarization ... #Article#Multi#PersonalizedDocumentSummarization#NLP#SearchEngine
Issue Date: 2017-12-28 WebInEssence: A Personalized Web-Based Multi-Document Summarization and Recommendation System, Radev+, NAACL, 01, 2001.06 Comment・ドキュメントはオフラインでクラスタリングされており,各クラスタごとにmulti-document summarizationを行うことで, ユーザが最も興味のあるクラスタを同定することに役立てる.あるいは検索結果のページのドキュメントの要約を行う. 要約した結果には,extractした文の元U ... #Article#PersonalizedDocumentSummarization#NLP
Issue Date: 2017-12-28 A Study for Documents Summarization based on Personal Annotation, HLT-NAACL-DUC’03, Zhang+, 2003, 2003.05 Comment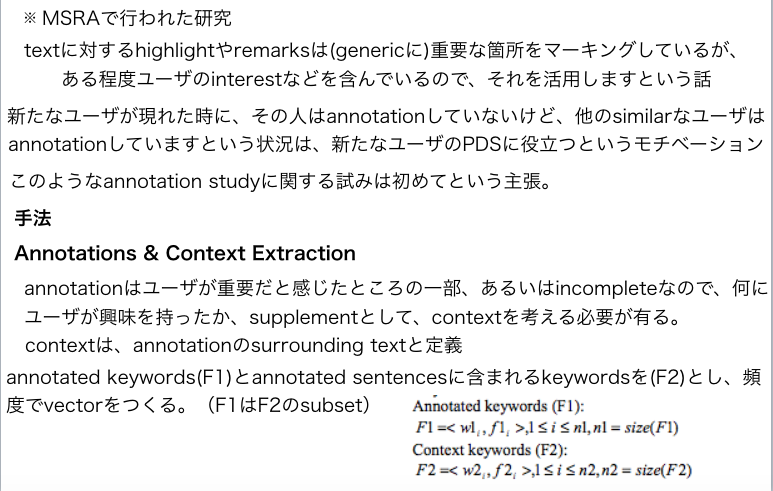 ![image](https://user-images.githubuse重 ...